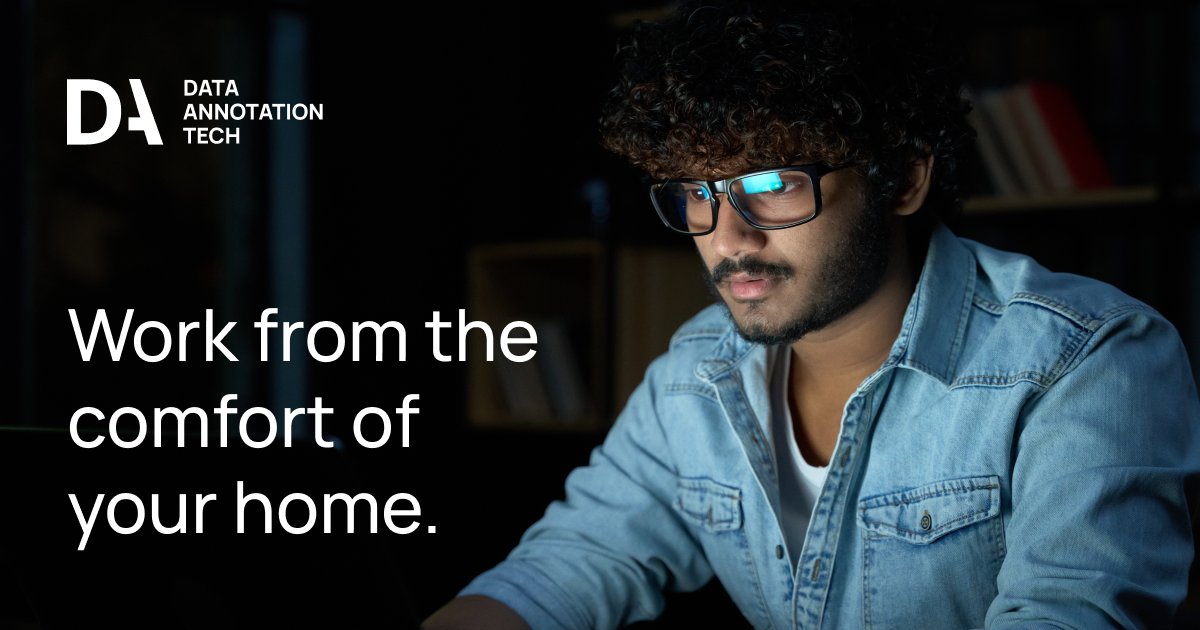
Data annotation tech? The key to efficient machine learning. Picture this: a world where algorithms learn from labeled data to perform tasks with precision. Imagine the immense potential this unlocks for various industries. From image recognition to natural language processing, data annotation tech plays a vital role in enhancing AI capabilities. Let’s delve deeper into the fascinating realm of data annotation technology. Let’s explore how it shapes the future of artificial intelligence. Are you ready to embark on this enlightening journey with us? Let’s begin.
Data Annotation Technology: Enhancing Machine Learning Models
Data annotation technology plays a pivotal role in the realm of machine learning, acting as the cornerstone for training algorithms and enhancing model accuracy. In this comprehensive guide, we delve into the intricacies of data annotation tech, exploring its significance, methods, challenges, and future trends.
The Importance of Data Annotation in Machine Learning
In the realm of machine learning, data annotation serves as a fundamental process that involves labeling data to provide context and meaning for algorithms. The annotated data helps models understand patterns, make predictions, and improve accuracy. The key significance of data annotation technology includes:
– **Training Machine Learning Models**: Annotated data forms the basis for training machine learning models, enabling algorithms to learn from labeled examples and make informed decisions.
– **Enhancing Accuracy**: Accurate annotations enhance the precision and reliability of machine learning models by ensuring that the algorithms are trained on relevant and correctly labeled data.
– **Facilitating Supervised Learning**: Data annotation is essential for supervised learning tasks, where algorithms are trained on labeled data to predict outcomes for new, unlabeled data.
– **Supporting Various Domains**: From healthcare to autonomous driving, data annotation technology caters to a wide range of industries and applications, providing customized solutions for diverse datasets.
Methods of Data Annotation
Data annotation encompasses various methods and techniques tailored to different types of data and machine learning tasks. Some common methods of data annotation include:
– **Bounding Boxes**: Used for object detection tasks, bounding boxes define the location and boundaries of objects within an image or video frame.
– **Semantic Segmentation**: This method involves pixel-level annotation, where each pixel in an image is labeled to differentiate objects or regions.
– **Classification**: Data is annotated with specific class labels to categorize information, facilitating classification tasks in machine learning.
– **Text Annotation**: In natural language processing (NLP), text annotation involves labeling text data for sentiment analysis, named entity recognition, and other NLP tasks.
– **Audio Annotation**: Audio data annotation includes labeling speech, music, or other audio signals to train models for speech recognition or sound classification.
Challenges in Data Annotation
While data annotation is crucial for machine learning success, it comes with its own set of challenges that can impact the quality and efficiency of annotated data. Some common challenges include:
– **Annotation Consistency**: Ensuring consistency across annotators is essential to maintain accuracy and reliability in labeled datasets.
– **Scalability**: Managing large datasets and scaling annotation processes to handle increasing volumes of data can be a logistical challenge.
– **Quality Control**: Implementing robust quality control measures is crucial to identify and rectify annotation errors that may affect model performance.
– **Subjectivity**: Annotating subjective data, such as sentiment analysis in text, can introduce bias and variability among annotators.
Future Trends in Data Annotation Technology
As technology evolves and machine learning applications become more sophisticated, the landscape of data annotation is also poised to witness significant advancements. Some emerging trends in data annotation technology include:
– **AI-Powered Annotation Tools**: Integration of artificial intelligence (AI) algorithms in data annotation tools to automate annotation processes and improve efficiency.
– **Active Learning**: Implementing active learning strategies to optimize data annotation by selecting the most informative samples for annotation, reducing labeling effort.
– **Multi-Modal Data Annotation**: Catering to the growing demand for multi-modal data annotation, which involves annotating data from multiple sources such as images, text, and audio.
– **Crowdsourcing Platforms**: Leveraging crowdsourcing platforms to scale annotation tasks and engage a diverse pool of annotators for large-scale projects.
In conclusion, data annotation technology serves as a foundational pillar in the realm of machine learning, enabling algorithms to learn from labeled data and make accurate predictions. By understanding the importance, methods, challenges, and future trends in data annotation, organizations can harness the power of annotated data to drive innovation and enhance machine learning capabilities.
Avoid These 10 Mistakes On DataAnnotation.Tech
Frequently Asked Questions
What is data annotation technology?
Data annotation technology involves the process of labeling data to make it understandable for machines. It helps in training and improving machine learning models by providing context and meaning to raw data.
How is data annotation done in practice?
Data annotation is typically done by human annotators who carefully label specific features in a dataset. This can involve tasks such as image segmentation, text categorization, sentiment analysis, and more.
Why is data annotation important in machine learning?
Data annotation is crucial in machine learning as it helps algorithms learn patterns and make accurate predictions. High-quality annotated data ensures the reliability and performance of machine learning models.
What are the main challenges in data annotation?
Some common challenges in data annotation include ensuring annotation consistency, handling large volumes of data, maintaining data privacy and security, managing annotator bias, and ensuring the quality of annotations.
What are the different types of data annotation techniques?
Various data annotation techniques include image classification, object detection, named entity recognition, sentiment analysis, audio transcription, and more. The choice of technique depends on the type of data and the specific machine learning task at hand.
Final Thoughts
In conclusion, data annotation tech is pivotal in enhancing machine learning models’ accuracy. Quality annotation ensures reliable training data, leading to improved AI system performance. Leveraging advanced annotation tools optimizes the annotation process, saving time and resources. Investing in data annotation tech is indispensable for organizations striving for AI excellence.