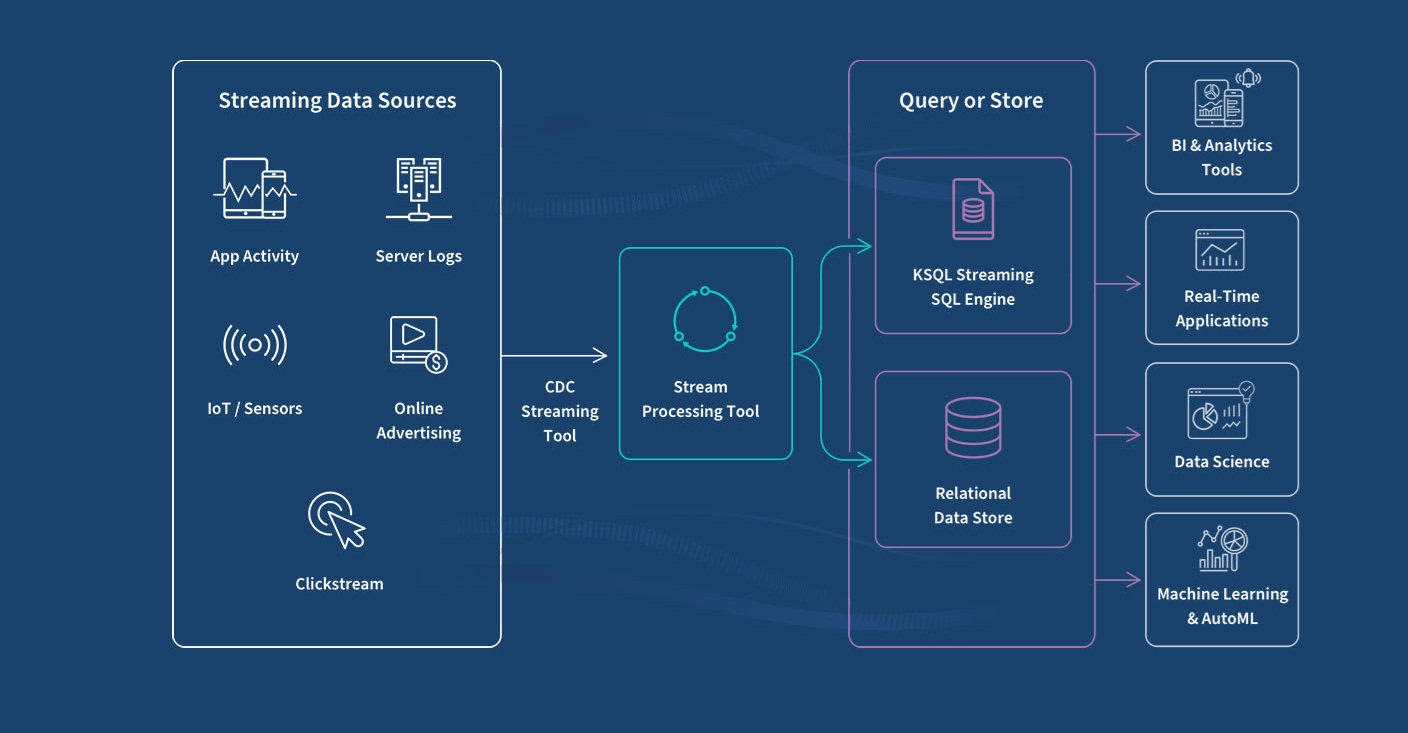
Introduction
The proliferation of Internet of Things (IoT) devices has revolutionised industries by enabling real-time monitoring, automation, and enhanced decision-making. IoT devices generate massive amounts of data, from smart homes to industrial automation. However, the real value lies in collecting this data and analysing and leveraging it effectively. This is where data science plays a crucial role, particularly in streaming data. For professionals aiming to master this field, enrolling in a Data Science Course can provide the necessary skills and knowledge to handle IoT applications effectively.
Understanding IoT and Streaming Data
IoT devices are embedded systems with sensors, actuators, and communication hardware that interact with their environments. These devices continuously generate streaming data—an ongoing flow of information transmitted in real-time or near real-time. Examples include temperature readings from smart thermostats, health metrics from wearable devices, and traffic data from smart cities.
Streaming data is characterised by its high velocity, variety, and volume. Unlike traditional batch data processing, which handles static datasets, streaming data requires real-time analysis. This immediacy is crucial for IoT applications where timely insights can lead to significant outcomes, such as preventing equipment failure or optimising resource utilisation. A comprehensive data course that follows an updated curriculum, such as a Data Science Course in Mumbai will include modules on handling streaming data, providing learners with a strong foundation to tackle such challenges.
Data Science in Streaming Data
Data science provides the tools, techniques, and frameworks to extract meaningful insights from streaming data. Key contributions of data science to IoT streaming data include:
Real-Time Analytics
Data science enables the real-time processing and analysis of streaming data. Algorithms and frameworks like Apache Kafka, Apache Flink, and Spark Streaming allow for data ingestion, processing, and analysis on the fly. These technologies empower IoT systems to make immediate decisions, such as detecting anomalies in machinery performance or adjusting traffic signals based on real-time congestion. Learning these technologies is often a key component of a modern Data Science Course.
Anomaly Detection
IoT devices often operate in dynamic environments where unexpected events can occur. Anomaly detection algorithms, such as isolation forests and deep learning-based methods, help identify irregular patterns in streaming data. For example, in predictive maintenance, anomaly detection can pinpoint signs of equipment malfunction, enabling proactive interventions and reducing downtime. These advanced analytical techniques are frequently detailed in any career-oriented data course, for instance, a Data Science Course in Mumbai that prepares professionals for real-world scenarios.
Predictive Analytics
Predictive models built using machine learning are integral to IoT applications. These models use historical and real-time data to forecast future events. For instance, energy companies use predictive analytics to optimise electricity distribution by predicting consumption patterns based on IoT sensor data. Mastering predictive analytics is vital in any well-rounded Data Science Course, ensuring practitioners can develop and deploy accurate models.
Data Fusion
IoT ecosystems often involve multiple devices generating diverse data types, such as video, audio, and sensor readings. Data science facilitates data fusion—combining data from different sources to create a cohesive understanding of the environment. This is essential in applications like autonomous vehicles, where data from cameras, LIDAR, and GPS must be synchronised for accurate navigation.
Edge Computing Integration
IoT devices often operate in environments with limited bandwidth or latency constraints. Data science supports edge computing by enabling analytics to occur directly on the devices or at the network’s edge. Techniques like lightweight machine learning models and on-device inferencing ensure real-time insights without relying on cloud processing.
Challenges in Streaming Data for IoT
While data science unlocks immense potential in IoT streaming data, several challenges must be addressed:
- Data Quality: IoT data often contains noise, missing values, or inaccuracies, necessitating robust preprocessing techniques.
- Scalability: The sheer volume of data from millions of devices requires scalable frameworks and infrastructure.
- Latency: Achieving low-latency processing is critical for real-time applications like autonomous vehicles or industrial automation.
- Privacy and Security: Streaming data may include sensitive information, raising concerns about data protection and regulatory compliance.
Applications of Data Science in IoT Streaming Data
Here are some applications that illustrate the use of data science principles in streaming data for IoT.
Smart Cities
IoT devices monitor traffic patterns, air quality, and energy usage in smart city initiatives. Data science enables the analysis of this data to improve urban planning, optimise traffic flow, and reduce pollution. For example, adaptive traffic lights use streaming data to minimise congestion by dynamically adjusting signal timings.
Healthcare
Wearable devices generate continuous data on vital signs, physical activity, and sleep patterns. Data science models analyse this information to provide real-time health insights, detect anomalies, and support telemedicine applications. For instance, early detection of heart irregularities can save lives.
Industrial IoT (IIoT)
IoT devices monitor machinery, supply chains, and environmental conditions in industries. Data science enables predictive maintenance, operational optimisation, and energy efficiency. For example, predictive models can anticipate equipment failures, reducing costs and downtime.
Agriculture
IoT-enabled sensors in agriculture monitor soil moisture, weather conditions, and crop health. Data science techniques analyse this data to optimise irrigation, improve crop yield, and reduce resource wastage.
Retail
Retailers use IoT devices to track inventory, monitor customer behaviour, and manage supply chains. Streaming data analysis helps optimise inventory levels, personalise customer experiences, and enhance demand forecasting.
Future Directions
As IoT continues to grow, the role of data science in streaming data will expand. Emerging trends include:
- AI and Machine Learning at Scale: Advances in AI will enable more sophisticated real-time analytics and autonomous decision-making.
- Federated Learning: This approach allows IoT devices to train machine learning models while preserving data privacy collaboratively.
- Advanced Edge Computing: Enhanced edge AI capabilities will reduce latency and reliance on centralised cloud systems.
- Blockchain for Data Security: Blockchain technology will significantly improve the integrity and security of IoT data streams.
Conclusion
The intersection of data science and IoT streaming data drives transformative innovations across industries. By enabling real-time analytics, predictive insights, and actionable intelligence, data science empowers IoT systems to operate efficiently and effectively. For those eager to contribute to this dynamic field, a Data Science Course can provide the expertise to effectively manage and analyse streaming data. As technology advances, the synergy between IoT and data science will unlock new possibilities, shaping the future of connected devices and smart systems.
Business Name: ExcelR- Data Science, Data Analytics, Business Analyst Course Training Mumbai
Address: Unit no. 302, 03rd Floor, Ashok Premises, Old Nagardas Rd, Nicolas Wadi Rd, Mogra Village, Gundavali Gaothan, Andheri E, Mumbai, Maharashtra 400069, Phone: 09108238354, Email: enquiry@excelr.com.